Duration: 09/09/2019 – 08/09/2023
Type of project: COST project
Chair: Isabelle Augenstein, Denmark
MC on UM FERI: assoc. prof. dr. Matej Rojc
Link: COST CA18231
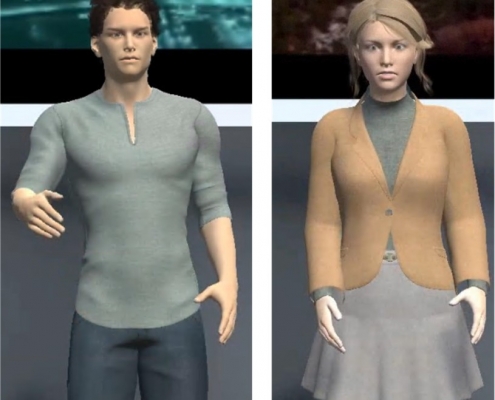
Duration: 09/09/2019 – 08/09/2023
Type of project: COST project
Chair: Isabelle Augenstein, Denmark
MC on UM FERI: assoc. prof. dr. Matej Rojc
Link: COST CA18231
Natural Language Generation (NLG) encompasses all Natural Language Processing (NLP) tasks that deal with automatically (or semi-automatically) generating readable text. Texts generated by NLG models usually have a large audience and can be quite application-specific. Those applications can be categorised in two broad areas: text-to-text and data-to-text generation. The former takes existing texts as input and produces a coherent text as output. Example applications include e.g. question answering (QA), converting nonlinguistic data to text, for instance, football commentary, weather and financial report generation, patient information summarization, and image/video caption generation.
Dialogue, interaction and conversational language generation applications
HCI through natural language conversation is currently one of the most active research areas, where new commercial applications are emerging virtually every day. The so-called artificial intelligence paradigm (in most cases deep learning) is often used in the dialogue-based problem solving. Although, at present, the achievements are promising and many global companies and universities have focused their HCI efforts, the challenges of truly intelligent natural language understanding (NLU), and appropriate NLG regarding response appropriateness and natural outputs have not yet been addressed. The CA18231 will go beyond state-of-the-art technology in this field, using LG models in HCI task interaction for many interesting and challenging real-world use cases, such as conversational search interfaces; grounded dialogue models; real-time dialogue models; and conversational robots. Due to the complexity of NLU and NLG, much research focuses on the development of end-to-end systems. However, these are highly dependent on the availability of relevant data for learning. The lack of the required dataset (especially multimodal ones) limits the deep learning research possibilities for HCI. CA18231 project will solve this problem by construction of multilingual multimodal datasets to evaluate multimodal conversational interfaces. The current methods have not yet solved the problem of having a truly natural conversation. One main issue here is the difficulty of evaluating NLG systems since intrinsic evaluation metrics (e.g. BLEU, ROUGE) are often a poor proxy for model performance (Belz, 2009). This CA18231 will address this issue first by stressing the need for extrinsic evaluation. Concretely, dialogue agents will be tested by industry members in controlled environments.
Project group members
Periodic meeting for COST action CA18231
1st MC MEETING – COST KICK OFF MEETING