Key research activities in the HUMANIPA project are focused on understanding and modeling conversational language in human-to-human interactions. In the field of natural language processing and understanding of spoken language, begins to play an important role in human-machine interaction gesticulation and non-verbal communication, as well as the ability to express information not only through words. Namely, it has become clear that integration of such signals represents a key direction in identifying of a more personal aspect of user inputs and how device responses can be presented to the people. In direct interaction, non-verbal signals transmitted along with spoken content (or even in the absence of it) are crucial for establishing cohesion in the discourse.
It could be said that verbal/linguistic parts of spoken language (e.g. words, grammar, syntax) carry a symbolic/semantic interpretation of the message, while co-verbal parts (e.g. gestures, expressions, prosody) carry the social component of each message and serve as orchestrator of communication. The main source of non-cohesiveness (negative perception) in response is precisely the lack of conversational knowledge. Such knowledge consists of communicative signals, linguistic, paralinguistic and even social domain that need to be explored in more detail. As part of the HUMANIPA project we, therefore, propose a study of spoken language as a multimodal extension of the spoken language, which includes non-verbal behavior (body language) in addition to speech. In this regard, we propose a multifaceted function that based on information fusion, which links natural language processing (NLP) and body language processing (ELP) into a unified conversational language understanding (CLU) system.
The conversational space proposed under the HUMANIPA project is extensive. Partial fusion functions covered by ELU (embodied language understanding) are cognitive and pragmatic in nature. The proposed paralinguistic signals (e.g. forms, prosody), and their propagation through dialogue are not subject to any well-defined grammatical rules. Generally established linguistics (e.g. text as a recognized sequence of words) does not directly contain much context that goes beyond semantic meaning, e.g. in terms of intent/meaning identification, it does not contain any deterministic markers for a specific intent. Such an input, therefore, does not allow direct and deterministic conclusions. The proposed ELU concept and research work, therefore, cover a wide range of tasks: analysis of multimodal corpora of human-to-human conversational behavior, context-independent prediction of forms and movements (motor skills), design of the so-called semiotic grammar and context-dependent lexicon of verbal behavior (gesticon), repositories of motor skills, linguistic resources, prosodic and linguistic nature of gestures and expressions, etc., as elementary components of the fusion functions.
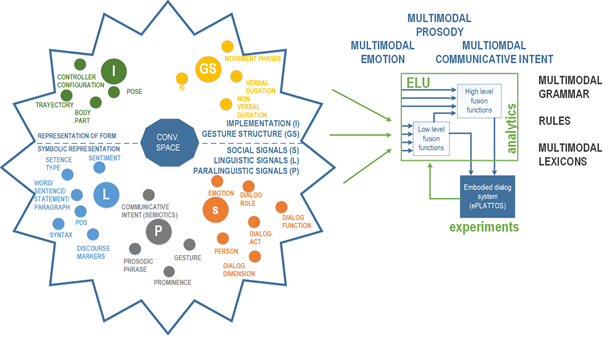
In general, the research work is based on approaches derived from natural language processing, big data analytics, statistical modeling, and machine learning. Thus, we investigate optimal inference (possible fusion functions) on the basis of evaluation with newly developed CLU algorithms. Based on the inquiry and descriptive research that will be conducted through the analysis of relevant conversation scenarios in spontaneous informal discourse, we will formulate hypotheses, conclusions and assumptions regarding the fusion functions (conversational concepts) in the conversational space. Through explanatory research, we will also explore multi-signal relationships and intertwining conversational concepts, thus designing new conversational strategies with associated verbal and non-verbal sources. So we will design i.e. conversational knowledge. Based on the conversational knowledge (hypotheses and conclusions), we will design new low/high-level fusion as artificial intelligence algorithms and test them through qualitative and quantitative approaches. This will turn conversational knowledge into actual interaction strategies and models. The information used as a learning material will include spontaneous conversations, with a particular focus on lectures and conversation settings among multiple participants. The algorithms will be developed and evaluated in appropriate environments (e.g. smart home and AAL), and with the appropriate public (adults, older adults, vulnerable groups, etc.).
Project phases and realization: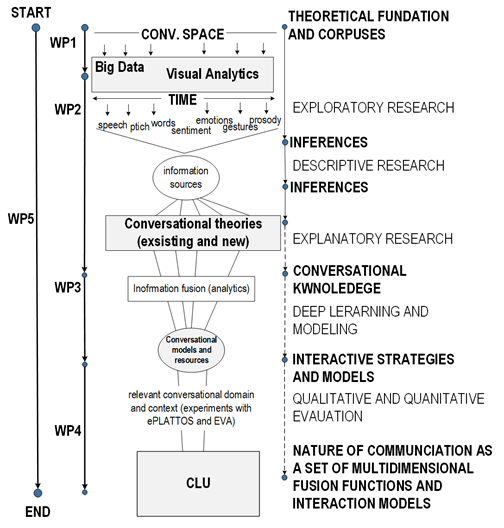
DP1: In this phase, we will establish the theoretical basis and justification for the CLU concept, and provide a common understanding and consistent specification of conversational contexts, domains, requirements and relevant theories in fields, such as: corpus linguistics, multimodal fusion, conversational agents (with body) and conversational behavior, program structure, and identification of key performance indicators and methods, and evaluation and validation metrics.
DP2: In this phase, we will discover and formulate hypotheses, conclusions and assumptions regarding the interaction of ‘basic’ conversational signals, how to integrate them into conversational concepts, and how these conversational concepts, together with the basic signals, interact with communication (i.e. multilayered concepts). The work will cover exploratory and descriptive research conducted through the use of experimental analysis of multimodal conversational corpora based on visual analytics conducted through dedicated analytical tools and a tool for conducting analytics over mass data. We will outline the most natural and optimal transformations of a complex conversational space into conversational artifacts (concepts), which are presented as orderly and evenly coded information sources connected via a common time axis.
DP3: In this phase, we will focus on the research and development of new deep-learning based fusion functions capable of modeling different levels of multimodal fusion, in order to identify and classify communicative intents in conversational episodes (open domain). We will harness the information sources, conclusions and data obtained from DP2 and the advanced deep learning frameworks, and translate conclusions and hypotheses from strategies into actual interaction models applicable in various fields and concepts in the HMI.
DP4: In this phase, the new models will model the concept of conversational dialogue, which is capable of conducting and leading complex human-machine interaction (dialogue) in an open and closed domain. The phase is also intended to quantitatively and qualitatively evaluate the new CLU paradigm, the identified conclusions, and the developed fusion functions in appropriate contexts and with the help of relevant participles. For the qualitative part of the experimental work, we will upgrade the relevant approaches of the perceptual experiments, and design more experiments with scenarios that target specific conversational concepts of a particular low or high level fusion function. For this purpose, we will use existing dialogue systems and ECA technology, and extend them with the ability to process, to identify and to classify communication purposes in target contexts. For each scenario, we will define a standardized taxonomy of valuation based on the Likert scale.
DP5: This phase is intended for project management, requirement specification, and dissemination.
Workshops and conferences
Researchers in the project
Publications